AI Assistant
Contextual AI scaling with complexity

AI copilot experience for a connected enterprise platform of media planning, optimization and administration applications
Overview
Role: Director of Product Design
Team: Product Design Team
Timeline: 2024-2025
Purpose
Purpose of the AI Assistant is to provide impactful support to user workflows as a complement to existing AI Console features across the Interact platform (platform used by IPG for end-to-end media lifeycle management). There were no pre-existing workflows and we needed to research, produce a proof of concept and scope designs for an AI Assistant MVP that would scale well across all products in the platform.
Team
Selected and led designers working on this effort
Michal Polak, Associate Product Design Director
Mariana Savitska, Senior Product Designer
Dominik Sfluvik, Product Designer
Oleksandr Ladieiev, Junior Product Designer
Process
Discovery & Ideation
- Led a series of whiteboarding sessions with product and design teams to align on AI integration opportunities
- Mapped out potential AI touchpoints across the platform to identify where the assistant could deliver the most value
Prototyping & Validation
- Designed early wireframes and prototypes for a "copilot experience" that introduced AI-assisted workflows
- Created a UI kit and flow diagrams to demonstrate scalability across different product areas
- Collected structured feedback from product teams to refine usability and technical feasibility
- Worked actively with designers to refine designs to get to an MVP
Prioritization & Complexity Assessment
- Provided insights to structure our feature roadmap, categorizing features by complexity
- Worked closely with engineering to evaluate implementation feasibility
Refinement & MVP Definition
- Finalized the MVP with designers, focusing on a floater window and contextual toolbar actions for seamless AI assistance
- Worked with designers to create a flexible and clear way to validate MVP with product, developing a scalable research template for highlighting key AI-driven workflows across all products
Discovery & Ideation
There had already been many discussions and ideas circulating across products organically about how to integrate AI Console capabilities into specific product workflows. To consolidate all the thinking relevant to AI Assistant, I led a series of discussions internally with the design team and with product collaborators to begin developing a point of view on how to integrate AI Assistant across all products.
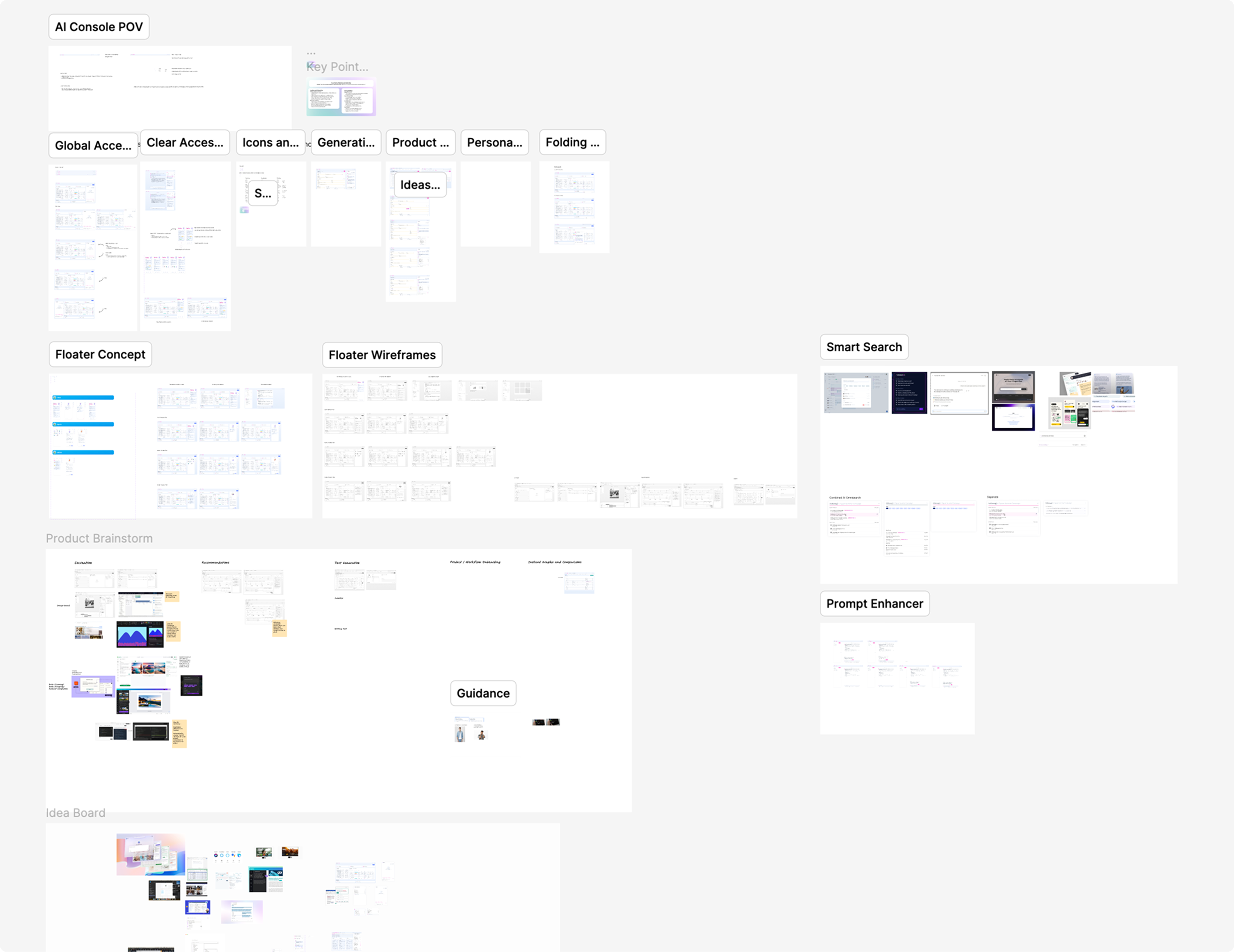
Proof of Concept
Since this was a project with no pre-existing workflows or clearly defined scope at the beginning (up to us to clarify and define), I take a hands-on approach in designing wires and initial proof of concepts for the designers to align and polish for the final MVP.
In parallel with discovery and ideation, I drafted up wires for what what was initially circulating as a "copilot experience".
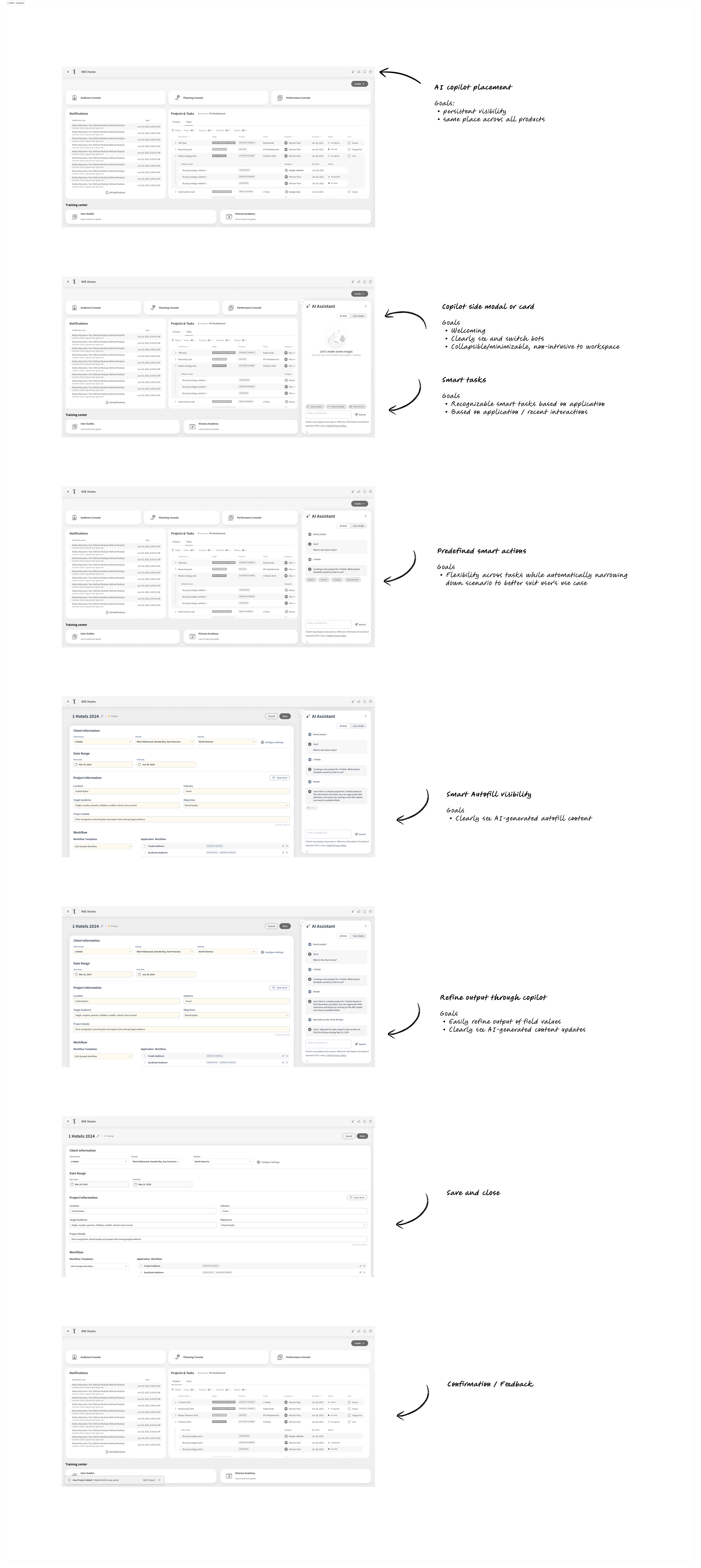
I also developed and designed the example UI kit, flow and prototypes for the proof of concept to circulate, share and gain more structured feedback across products on the experience and scalability
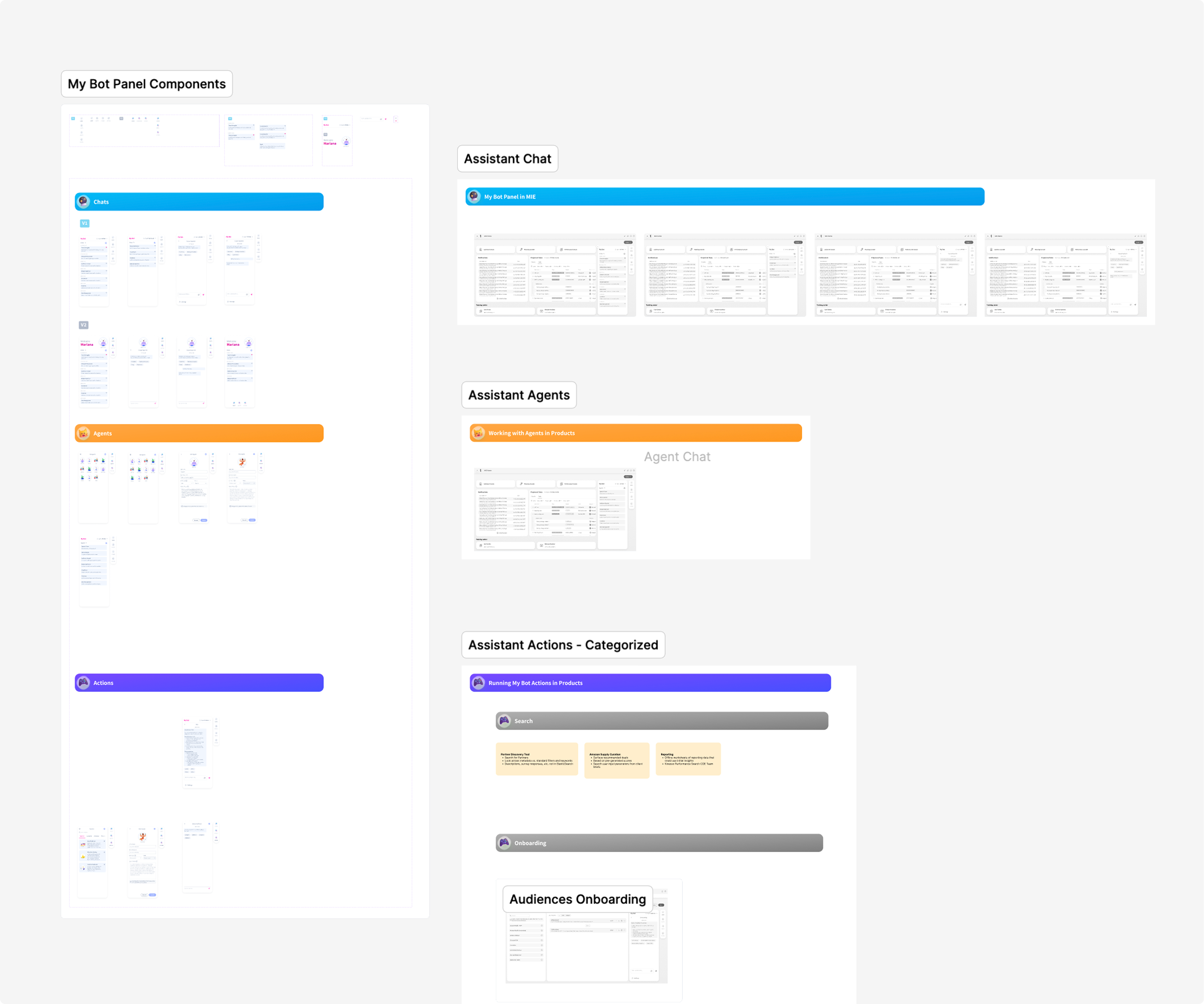
Product Sketches
Once the initial proof of concept began to take shape, I worked actively with designers to refine the design and created a template for how it would hypothetically be scaled across all products. We wanted to test the reaction of this experience across all product groups in the platform, so we used this as a template to gather input on usability, scalability, and ideas for how the AI Assistant would support product workflows.
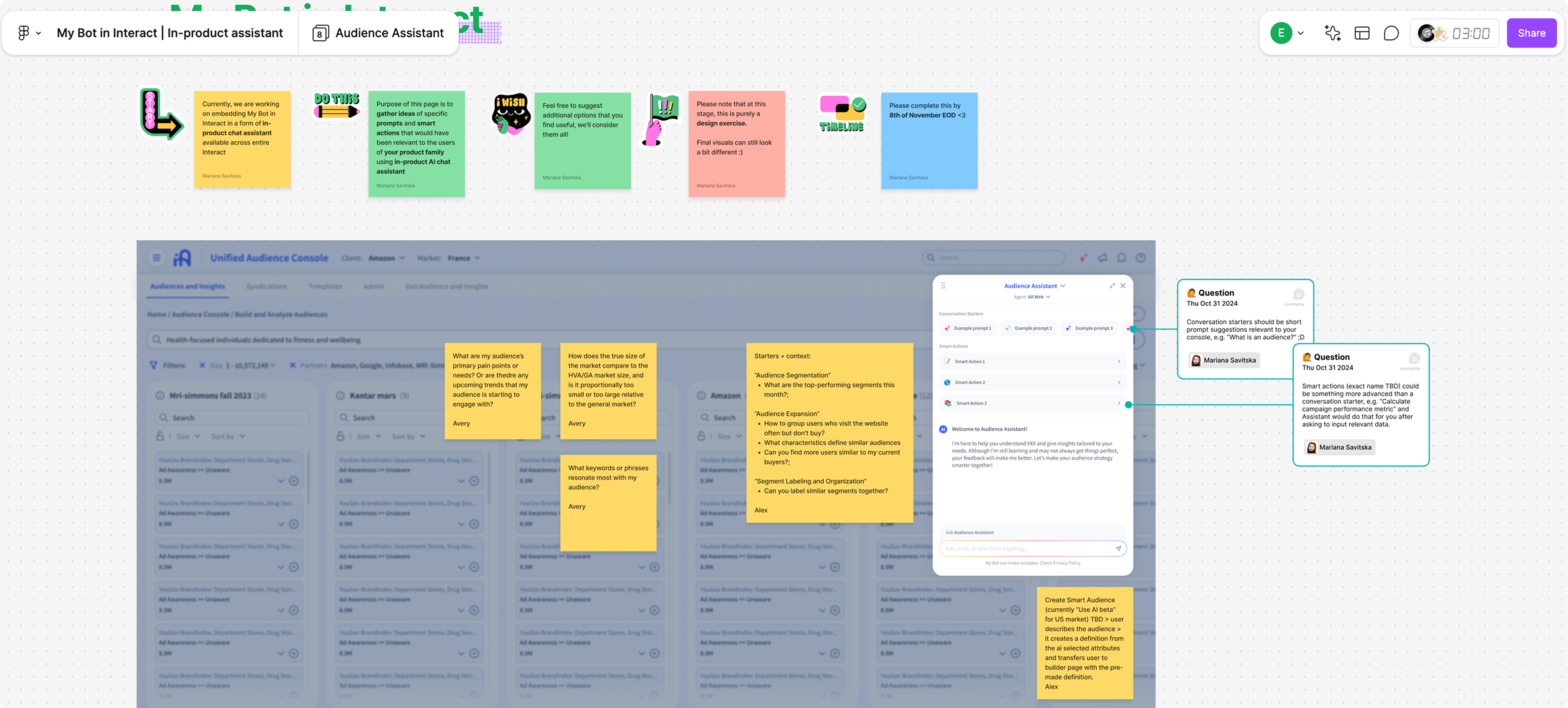
Prioritization
By MVP Scope
As we began to see common priorities and key areas of impact take shape, we began to first focus the AI Assistant MVP on the "floater window", and contextual toolbar actions such as running smart actions on interaction with the interface second.
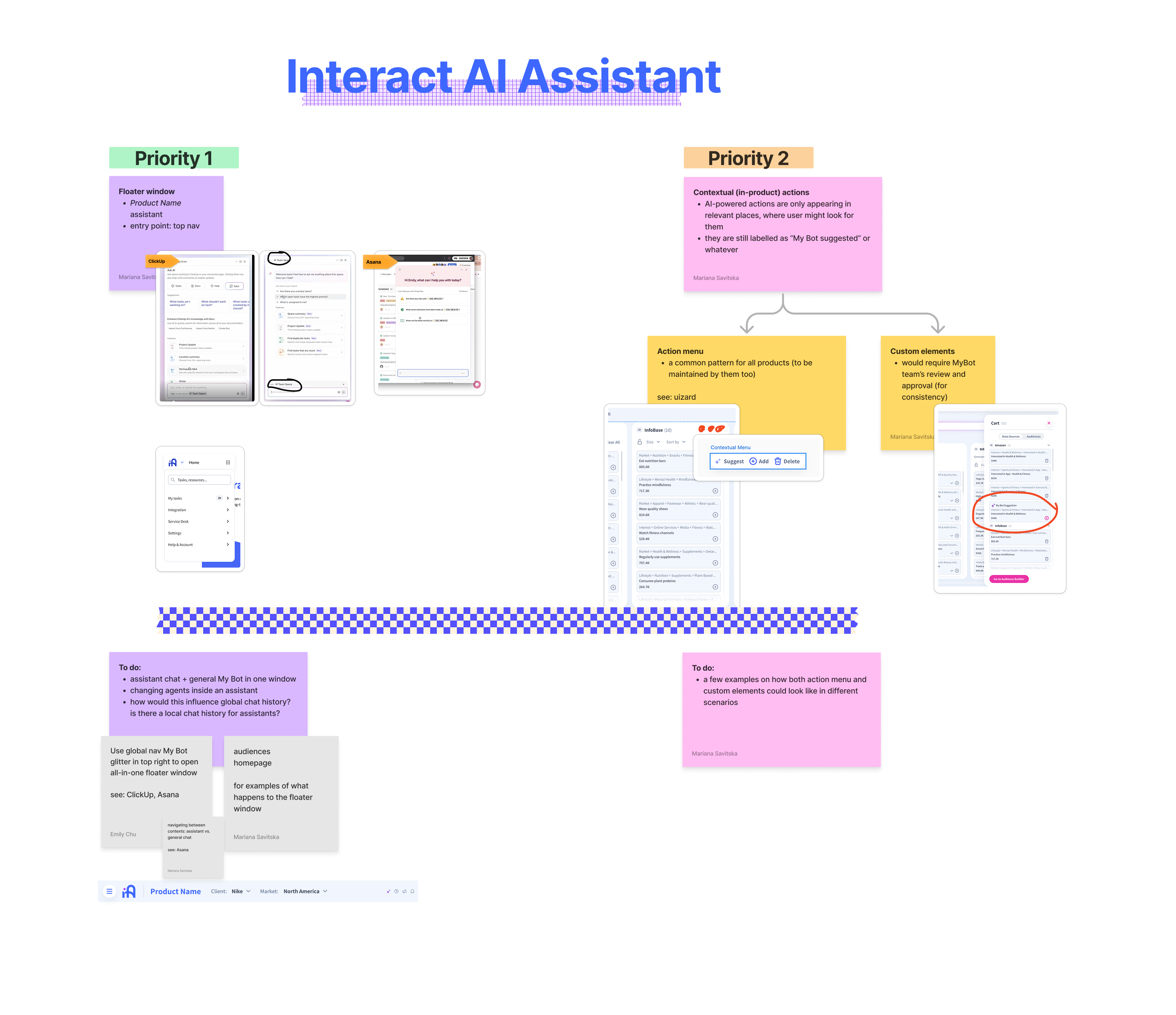
By Complexity
To share and begin evaluating build around the key priorities we were seeing emerge across all collaborators from the initial product sketches with engineering, I put together a summary of key ideas by complexity.
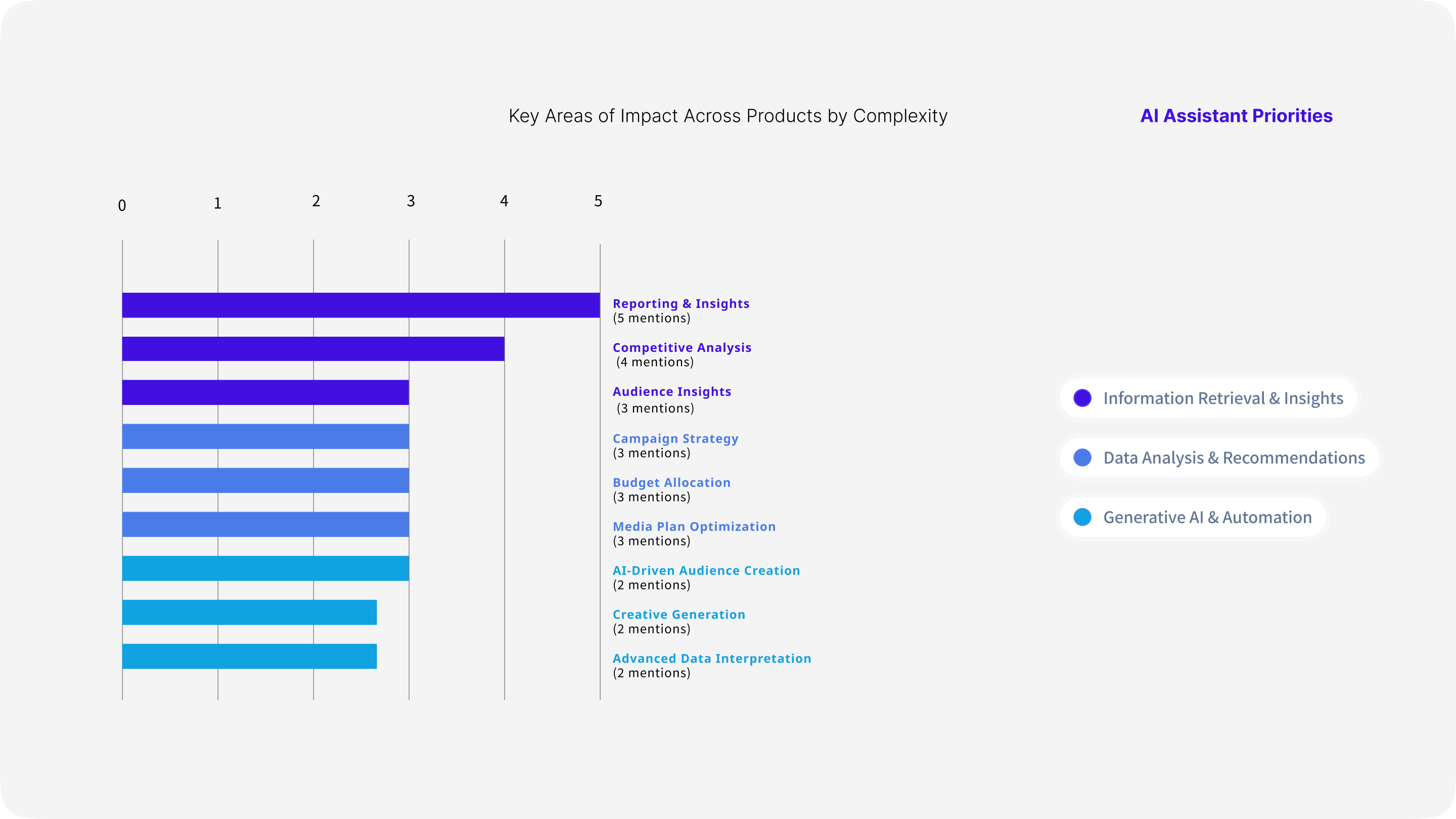
Lower Complexity
Information Retrieval & Basic Insights
Tasks focused on quickly retrieving and displaying relevant information using pre-defined rules and structured search functions. They require minimal AI customization and are easy to implement using existing data sources. Examples include:
Competitive Analysis
Fetching competitor spending and activity insights
Identifying strongest competitors in a given industry
Displaying performance benchmarks based on industry data
Reporting & Insights
Automating campaign performance summaries and reports
Providing structured insights on media investments
Generating quick responses to common analytics questions
Audience Insights
Identifying top-performing audience segments
Understanding customer pain points based on engagement data
Highlighting emerging trends based on audience behaviors
Medium Complexity
Data Analysis & Recommendations
Medium complexity tasks involving data analysis, identifying patterns, and providing actionable recommendations. Examples include:
Campaign Strategy
Assisting in campaign brief development
Recommending key messaging elements based on past success
Identifying target audience characteristics for optimized outreach
Budget Allocation
Suggesting budget splits across different media channels
Adjusting allocations based on performance and pacing trends
Recommending shifts in investment to maximize ROI
Media Plan Optimization
Recommending best-performing channels based on campaign goals
Exploring different media mix scenarios to optimize impact
Identifying inefficiencies in media spend and providing corrections
Higher Complexity
Generative AI & Advanced Automation
These tasks involve higher levels of AI-driven creativity, automation, and decision-making. They require significant customization, training, and integration with advanced/larger data sources. Examples include:
Creative Generation
Generating images based on text prompts (e.g., "Create a 300x250 image of a car in the jungle")
Producing brand-compliant creative templates for awareness campaigns
Suggesting ad copy based on brand tone and campaign objectives
AI-driven Audience Creation
Automatically generating audience segments based on user inputs
Suggesting lookalike audiences similar to existing customers
Identifying market gaps based on engagement patterns
Advanced Data Interpretation
Providing human-like interpretations of complex data reports
Enabling intelligent semantic search for emerging trends
Offering deep investment insights and performance tracking
MVP Designs
For the initial MVP, we decided to focus on an extension view of the AI Assistant: a context-aware, on-demand assistant accessible via a persistent top navigation extension across the Interact platform. It enables users to interact with AI-powered insights and actions without disrupting their workflow.

Key Features
On-Demand Accessibility
Users can access the AI assistant at any time via the top navigation
The floater window overlays the interface without persistent screen real estate usage
Context-Aware Interactions
Detects active workflows and provides relevant AI-powered assistance
Suggests smart actions based on the user’s current task
Quick AI Responses & Smart Actions
Users can input queries or select from AI-suggested actions
Supports information retrieval (e.g., campaign insights, competitor benchmarks)
Automates common workflows, such as summarizing reports or generating media plans
Adaptive UI & Seamless Integration
The interface dynamically adjusts based on the user’s location within the platform
Designed for non-intrusive, efficient interactions without disrupting ongoing tasks
Scalable for Future Enhancements
Built as a modular system, allowing for expansion with more AI-driven capabilities over time
Next Steps
User Testing & Iteration: Conduct usability tests with our users to validate impact and direction
Refinement of MVP Capabilities: Expand initial MVP capabilities to provide contextual recommendations and deeper analytical insights
Technical Implementation: Partner with engineering to define a structured implementation plan and identify use cases for priorities
Scalability Roadmap: Explore long-term AI integration strategies to support future product direction